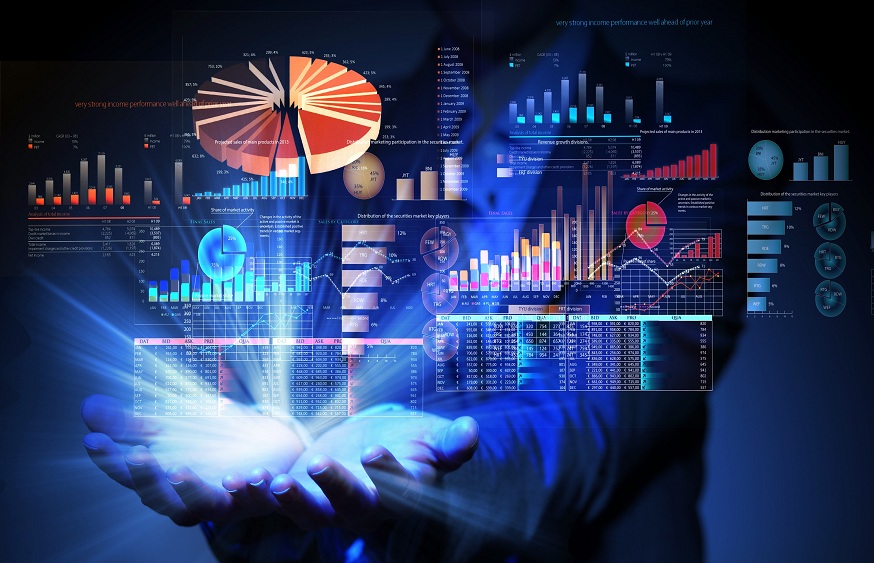
Best practices for ensuring data quality
Data is a value chain on its own. Over its entire life cycle and in all aspects of its operation, its impact on the activity of organizations continues to grow. An essential part of your Data Governance strategy , managing the quality of your data will require constant commitment and the establishment of a data-centric organization.
Whether it is for the success of your overall strategy, your complex use cases or that of recurring operational activities, the quality of data is critical in essence . Harvard Business Review estimated in 2017 that a task carried out with erroneous data incurred a cost 100 times greater than that of a task carried out using initially verified and correct data .
According to the 2020 Gartner analysis of data quality management solutions , more than 25% of the largest companies’ critical data is in error, to the point that the average cost of poor data quality could amount to 11M€ per year for organizations. The economic repercussions, positive or negative, must therefore be considered with the greatest attention.
New call-to-action
-
How to assess the quality of data?
The quality of data is measured through its intrinsic characteristics, whether internal or external to the company. We can cite the accuracy, completeness, consistency, validity, timeliness, integrity, clarity or even security of the data.
Data quality can be degraded at two levels. First of all at the level of the description of the data with, for example, conflicts between names of objects or imprecisions on the definition of objects. The other level is that of the data itself: null values, duplicates, abnormal values, obsolete data, etc.
The definition of poor quality data:
Inaccurate : missing information, incomplete, wrong numbers, spelling mistakes…
Non-compliant : by its nature or form, the data does not comply with the legislation or standards in force.
Uncontrolled : insufficient monitoring is carried out on the data which can duplicate with another or deteriorate over time.
Insecure or unreliable : if no control is applied, data becomes targets for hacking due to its vulnerability.
Static : Data that is not refreshed becomes stale and loses its usefulness.
Dormant : without updating or sharing, data becomes dead weight in your repository and no longer has value.
Aware of these pitfalls to avoid, the majority of companies have already tried to set up a data management process incorporating good practices and data quality measurement and control techniques.
However, these data management processes often remain compartmentalized, respecting the existing organizational silos and are not transverse to the functioning of companies. Only an organizational approach can improve data quality , by adapting investments to the business challenges related to these quality issues.
This approach is based on 4 pillars
The data quality management process should be iterative, relying on a “by design” data quality upgrade to a standard quality level defined in the data strategy. It will therefore be necessary to define upstream non-quality prevention processes as well as downstream anomaly remediation processes, not to mention data integrity checks.
In sum, this approach involves more planning in your data quality management. Without this, the value of your data assets will decrease and this will impact your operational activities.
-
Steps in the data quality management process
Four main steps are necessary to set up an effective data quality management process:
To this end, data lineage will help maintain data quality over time , by organizing the distinction between supplier and consumer processes. This is a data mapping, a real asset of the Data strategy, which ensures risk control and generates operational gains.
The lineage data will provide information on the origin, the successive treatments applied and the different end uses applied to the same data. The data can therefore be traced and its accuracy can be justified. This is the pillar of the data quality strategy.
-
Who are the guarantors of data quality?
3 key roles must be defined in your organization to guarantee the implementation of data quality, in accordance with the challenges of the company and the needs of exploitation by the business lines:
The Chief Data Officer
The Chief Data Officer (CDO) defines the rules of governance, monitors implementation and coordinates all Data Management activities.
The Data Management Executive (DME) organizes data management services, seeking to develop sharing and reuse for greater efficiency and consistency.
Guarantee the implementation in the IS of controls allowing compliance with quality requirements and regulatory constraints.
Monitor the costs related to Data Management on its scope and propose optimizations.
-
Choose your data quality management tool
The rules for quality assurance are of course specific to each business, but they must obey the main principles of data strategy and the processes that sustain quality assurance work .
According to the experience drawn from our missions at our customers, the internal development of a solution of management of the quality of data is not the most effective nor the most profitable way . Indeed, the functionalities to be implemented are numerous and complicated to maintain. It is preferable to turn to the products offered by software publishers specialists in the field
The choice of a data quality management tool requires first mapping the uses and functionalities to be covered by the solution, then evaluating the selection criteria: richness and relevance of the functionalities, price, durability of the solution, user experience, integration into the IS , training, quality reporting, etc.
-
Device for maintaining data quality
Maintaining data quality requires implementing good KPIs to measure: